How the EU’s recent or upcoming policies can help Europe to catch-up with US and China in AI diffusions
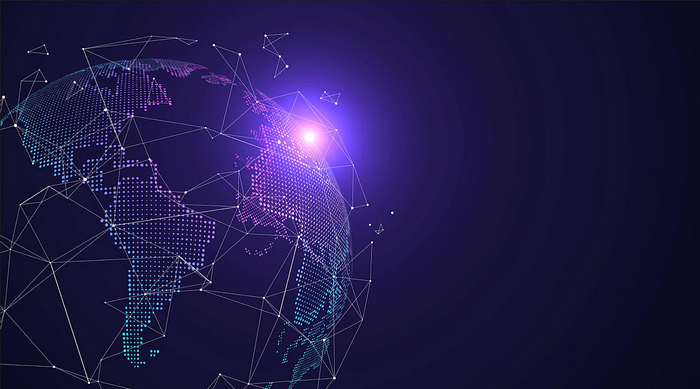
1. Executive summary
In terms of AI competitiveness, the European Union (EU) lags behind China and the U.S. Thierry Breton, European Commissioner for Internal Market, acknowledged in September 2020 that the EU missed the first wave of AI on personal data. (Kelly, 2021) This report addresses how the EU can catch up in AI against China and the U.S. My consulting project analysed six gaps in AI diffusion which are missing in the EU in comparison to China and the U.S through secondary research and expert interviews. Based on these “missing elements”, I derived seven recommendations on how to bridge the gap in AI diffusion in Europe. Finally, I synthesized the key learnings of the most important “missing element” — the data-sharing platforms — into three data platform archetypes. The answers are critical in the light of the global AI race where the EU must act today to become more competitive in artificial intelligence against China’s and the U.S.’ AI hegemony. To catch up in AI, the European governments, European industries, and tech giants1 should set up data platform orchestrators, build strategic partnerships with digital giants, collaborate globally on AI training, upskill and reskill AI talent, offer basic AI training, and encourage EU-based funding. These actionable insights are set out to influence policymakers and business leaders in Europe to create a win for all stakeholders in the AI race. By analyzing the EU’s gaps in AI and providing recommendations on how to catch up, the project helps Europe to achieve global AI competitiveness.
2. Introduction
It is established from findings of BCG (Francois, Burkner, et al 2020) that the European governments, EU industries, and tech giants are in a lose-lose-win relationship in the field of data and AI and this impacts AI diffusion in Europe and weakens Europe’s global AI competitiveness.
My research question considered the elements accelerating the gains in US and China as well as how EU policies could help diffuse AI. The outcome would also help my client, one of the worldwide leading strategy consulting firms and their clients understand the latest regulations and implications on their business.
The hypothesis led to postulating three missing elements influencing the lack of AI diffusion in the EU and these include lack of data, low funding, and relative weakness to train, retain talents as well as attract top talent.
Therefore, the goal of my project was to explore the factors causing the lose-lose-win as well as pose solutions to accelerate wins (see Fig. 1) for all stakeholders.
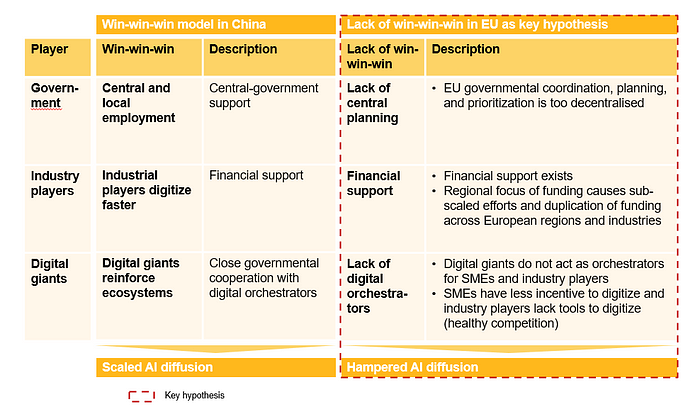
3. Research methodology
Given the nature of the research question, a qualitative research approach is found to be more relevant. This includes secondary research such as reviewing articles and reports, as well as qualitative interviews with experts. 12 experts from academia, consultants, and tech-industry were interviewed (see Table 1).
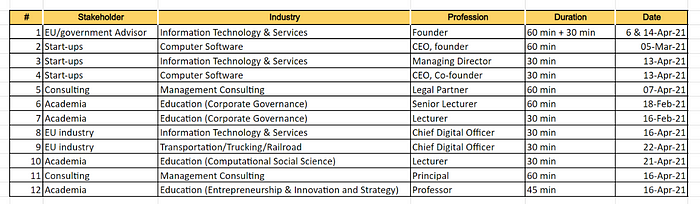
Given the time constraints, the research topic was broad and a degree of constriction should be necessary. The client however preferred not to prematurely withdraw opportunity reading insights in different elements. The client met my project team weekly to narrow down the scope and decide areas for further research based on the latest findings and the client’s commercial interest (see Fig. 2).
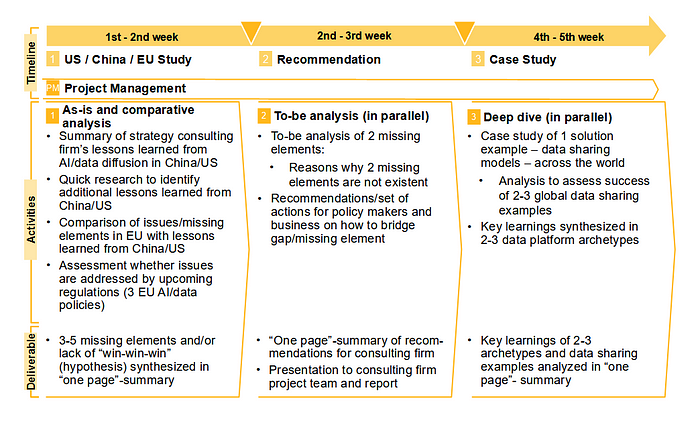
4. Analysis
To answer the question of how the European Union can catch up with AI diffusion against China and the U.S., the project team divided the analysis into three phases (see Fig. 3): comparative analysis, to-be analysis, and deep dive.
In the first analysis phase, the team compared what AI policies are missing in the EU with the lessons learned from the AI diffusion in the U.S. and China to identify three to six gaps or “missing elements”. In the second phase, the team derived seven recommendations for policymakers and leaders on how to bridge the gap in AI diffusion in Europe to provide actionable insights. In the third phase, the consultants selected the most important “missing element” in the EU and analyzed three global data sharing platform examples. The team synthesized their key learnings into three platform archetypes to generalize the findings.
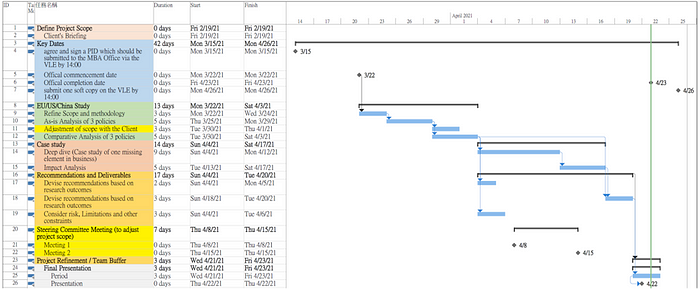
My team sees “missing elements” (see Fig. 4) that may discourage AI diffusion and eventually the client selected six elements around the topics data sharing, talent, and funding, for further study and chose a case about data sharing.
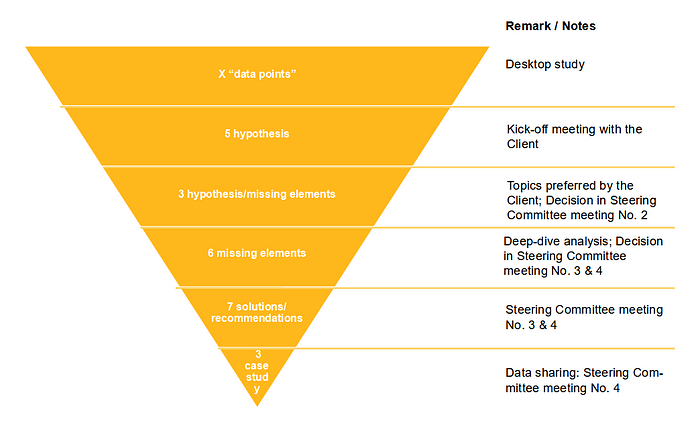
To analyze the lack of a win-win-win in the EU, the team derived five key hypotheses (see Fig. 5): access of data, talent and education, healthy competition, funding, and focused industries. The selection of the hypotheses was based on the learnings from the comparison of the AI diffusion in the EU with the U.S. and China.
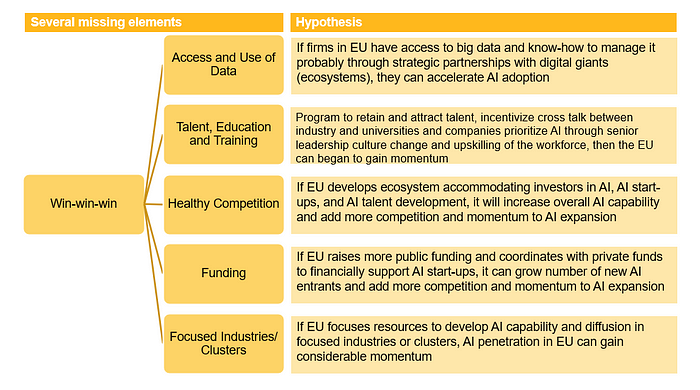
4.1. Comparative analysis
Castro, McLaughlin, and Chivot (2019, 2021) examined six categories of metrics — talent, research, development, AI adoption, data, and hardware to measure the AI progress in the economies of China, the EU, and the USA. Standard indicators to compare AI development among nations are not available. However, this study provided comprehensive metrics with transparent methodologies.
Overall, the EU ranks the last and the U.S. is leading. The EU leads in no category and is at second in talent, research, development, and adoption. The EU is the worst in data and hardware. China is in the leading position in AI adoption and data.
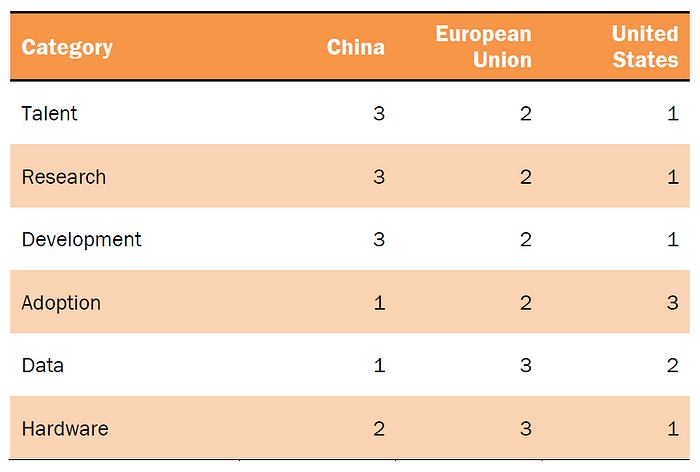
Talent is the entry point of AI development. The EU can produce a significant number (43,000+ in 2017) of AI talents (Castro, McLaughlin, and Chivot, 2019). However, talents frequently leave for U.S. firms. Keeping talent within the EU is a priority topic.
Data is a weak link for the EU. The EU owns massive public data but cannot use it widely to train AI. The General Data Protection Regulation, effective in 2018, has put constraints on the collection and use of data (Castro and McLaughlin, 2021).
4.2. To-be analysis
Drawing from the comparison, we are examining different options and approaches to help EU policymakers to accelerate AI diffusion in the EU. The options are derived from public articles and also expert interviews.
For actions on data, the team examines two general approaches: to establish a data ecosystem or to leverage the use of off-the-shelve pre-trained AI models. A data ecosystem promises to unlock the innovative potential of big data while a pre-trained AI model is believed to be an effective “black box” solution that helps accelerate the use of AI rapidly.
To cope with AI skill gaps, there are two common approaches: programs led by the public sector or by private companies. The government-led initiatives target a large pool of population and can cooperate with a high number of institutions and training centers in the country while company-led initiatives have gained momentum in recent years.
4.3. Case study analysis
Based on the derived solutions on how to bridge the gaps in AI diffusion in the European Union from the second phase, the team selected the most important “missing element” to make a deep dive into global examples (case study analysis). The goal was to identify different archetypes of data sharing models worldwide and generalize them in the form of key learnings. The synthesized platform archetypes serve policymakers and business leaders as a state-of-the-art approach to how a successful platform could be set up.
First, to analyze the data sharing examples, the team used an analysis framework with five elements: objective, stakeholders, must-haves, outcome, and risks. Second, to synthesis the lessons learned from the examples into archetypes, the researchers analyzed two elements — the success factors and pitfalls of the different models. To map the archetypes, the team build a fmy-field matrix with two dimensions — control and curation of the platform.
5. Results
In the comparative analysis, the team derived the main result that the AI diffusion in China and the U.S. is more mature than in the European Union.
The additional findings of the six “missing elements” for AI diffusion addressed the key question on how the EU can catch up in AI. Since the six “missing elements” comprised the access to data, AI talent and education, and AI-focused funding, the results built the basis for the recommendations to the sub-level research questions on how to democratize the access to data, accelerate funding, and influence upskilling and corporate education (Phase 2).
The results aligned with the project’s goal to assess the opportunities to turn around the current lose-lose-win relationship because they built the foundation to derive solutions for the acceleration of the AI diffusion (see Chapter 7).
5.1. Data
The first finding of the comparative analysis is the lack of access and usage of data to develop and deploy AI applications in the EU. The analysis showed that the lack of data is caused by the missing of data platform orchestrators and data ecosystems in the EU.
5.1.1. Data platform orchestrators
The first “missing element” which is hampering the AI diffusion in the European Union is the lack of data platform orchestrators to access interoperable and portable sector datasets.
The findings showed that the EU lacks a platform orchestrator to coordinate the data sharing across multiple industry stakeholders. In the EU, the situation is that the amount of available data is relatively small and fragmented across individual stakeholders in comparison to China or the U.S. (Expert interview CEO/Founder, Start-ups, 2021) Since these individual stakeholders fear technical, public relational, legal, and competitive risks, they are reluctant to cooperate on a platform.
To prove the impact of data platform orchestrators, the team analyzed the AI and healthcare sector in China (Zhang et al., 2018, p.j5910). The example showed that in China the Government acts as a platform orchestrator to promote precision medicine. For this purpose, the government supports centralized and integrated data platforms. Remarkable is that the data is collected from multiple stakeholders — such as the Government, hospitals, research institutes, industries, users — and integrated on platforms to perform data analysis.
5.1.2. Data ecosystem
The second missing element is that digital giants are unwilling to strike strategic partnerships with the EU governments and industry players (see Fig. 6). In the EU, the industry players lack AI know-how and data to train AI models. Strategic partners would collaborate to share tools, learning, and data, and help diffuse AI. However, there is a lack of a strategic partnership in the EU.
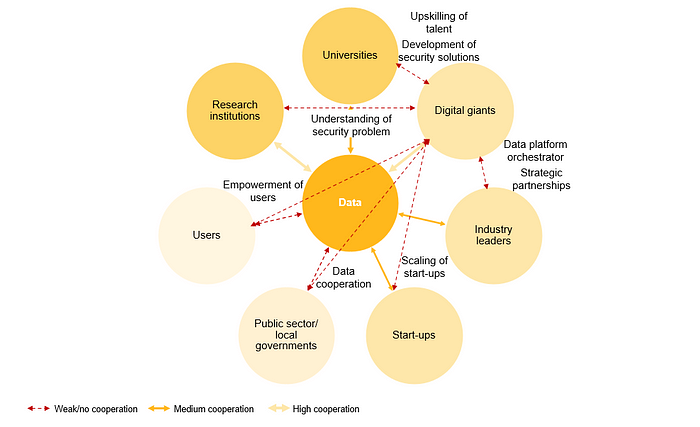
For example, the AI advisor Wolfgang Faisst argued that digital giants do not share tools and platforms to transform industry players into data-driven companies; rather they build partnerships only to ensure that the “industry brings data to utilize their clouds” (Expert interview Dr. Wolfgang Faisst, 2021). In another example, Google CEO Sundar Pichai commented on the idea of the open data ecosystem (Waters, 2020). While the EU plans to promote competition by forcing tech giants to open up their data to competitors, he recommended that “governments need to think through these important principles. Sometimes we can design very open ecosystems, they can have security implications.”
The relationship between digital giants and industry players is different in China. For example, Alibaba uses its data ecosystem to support its strategic partners to find temporary job-seekers during COVID (Chan et al, 2020). Alibaba’s open ecosystem enabled three local companies to find people furloughed by businesses affected by the pandemic.
With the new European Commission’s AI rules (European Commission, 2021), the EU will regulate AI to a greater extent, praising it as the “third way” between China’s state-led and the U.S.’s light-touch approaches. In contrast, it would be recommendable to follow the Ex-Google CEO Eric Schmidt’s argumentation (Heikkilä, 2021) that the EU should partner with large tech companies.
5.2. Talent
Both China and the EU are losing top AI talents and the talents drain to the USA. A study of authors who submitted academic papers to NeurIPS, which is a top conference in AI and accepts papers selectively, found that almost three-fifths (see Fig.7) of authors are working in the USA (MarcoPolo, 2021). On the other hand, MarcoPolo (2021) discovered that 18% (see Fig.8) of authors are from the EU, but only 10% (see Fig.7) are still working in the continental. China in retaining talents also performs no better than the EU.
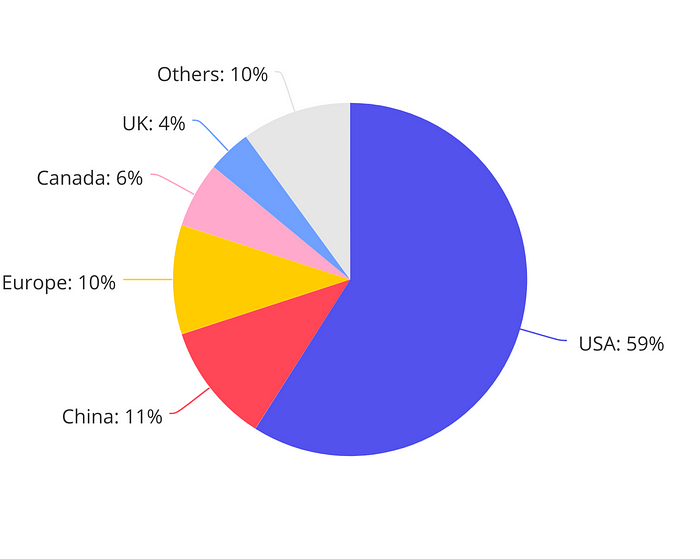
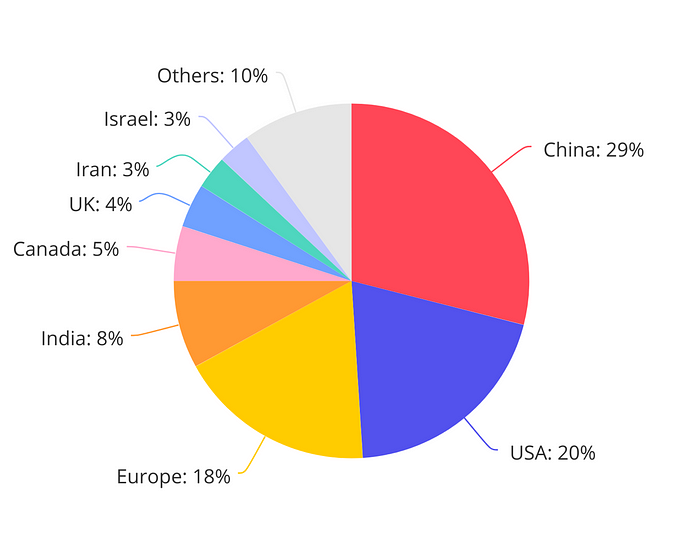
5.2.1 Talent Attraction
Universities play a strong role to supply talents while adapting the right curricula to cope with the rapid change is challenging (Benini and Nardelli, 2020). Benini and Nardelli (2020) see that boosting academic and industry collaboration enables academics to deliver up-to-date teaching. Freitas et al (2020) found that university-industrial collaboration has benefits for innovation diffusion. An expert (#12) in an interview stated that the fragmentation of administrative power within the university forms a hurdle for such collaboration. Another expert (#11) noted that some countries, such as France, cannot receive funds from private firms.
While Universities-industry collaboration may be challenging, EU stakeholders could include talents from the global pool. Talents from other countries can be a blue ocean for talent attraction. At the same time, EU Universities can approach overseas firms for partnership. LG, a South Korean multinational electronics company, can serve as an example.
LG partners with the University of Toronto to establish an AI Lab (University of Toronto, 2018). LG chose the University not only because the University can produce AI talents, but also LG has an AI Lab in Silicon Valley in California. Toronto is an extension (Mouncer, 2018). The Lab provides functions, such as R&D and training, and produces patents and publishing (Graham, 2020). The Lab in the opening was led by Dr. Graham, an expert in artificial neural networks, with extensive connections within Canada’s academia and business communities (Cho, 2019). The research teams mix with university students and a specialist from LG (Sattarzadeh, 2021; Plataniotis, 2021; Jang, 2021). Recently, the Lab has developed to solve the “black box” problem of AI a new “Explainable” artificial intelligence (XAI) algorithm, which outperformed comparable approaches on industry benchmarks. The finding is transferable to other industries and technology.
5.2.2. Upskilling/reskilling
Digitalization is transforming the nature of the employment relationship and creating the resultant effect of automation causing job replacement and displacement.
To prevent unemployment and increase productivity, policymakers and business executives in Europe must critically assess and adopt solutions that will accelerate upskilling of the corporate workforce in technologies that can aid in the digital transformation jmyney. Cedefop (2019) revealed that 70% of EU workers require at least moderate digitals skills to do their jobs while 1 in 3 of EU workers is at the risk of the digital skills gap. Therefore, upskilling employees in the knowledge of data science and digital technologies such as AI and its applications on jobs can aid AI diffusion.
5.2.3. EU population education
There are pockets of successes in nation wide education in digital technologies across Europe but more effort needs to be done. For instance in Finland, there is communication of basic understanding of AI for all citizens and the same approach in Latvia. This at scale effort is missing across many other countries and it is impacting AI diffusion.
5.3. Funding
The sixth element is that EU AI start-ups have less funding. AI investment in the EU is less than 2% of global AI funding in terms of funding share (Gartner 2018).
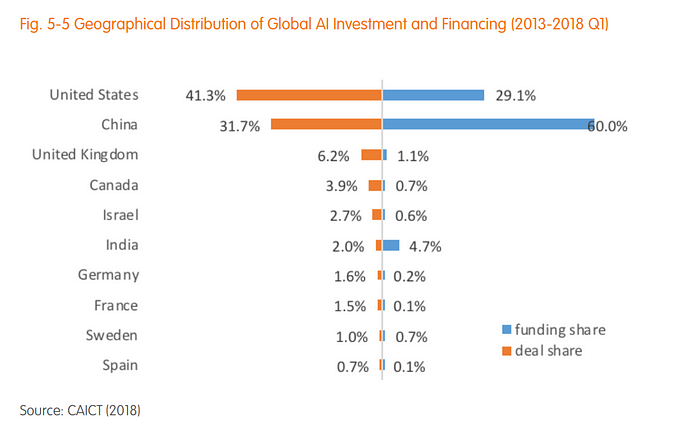
Most of AI start-up funding came from entities outside the EU. The EU investors preferred to invest in the early stages with small funding required.
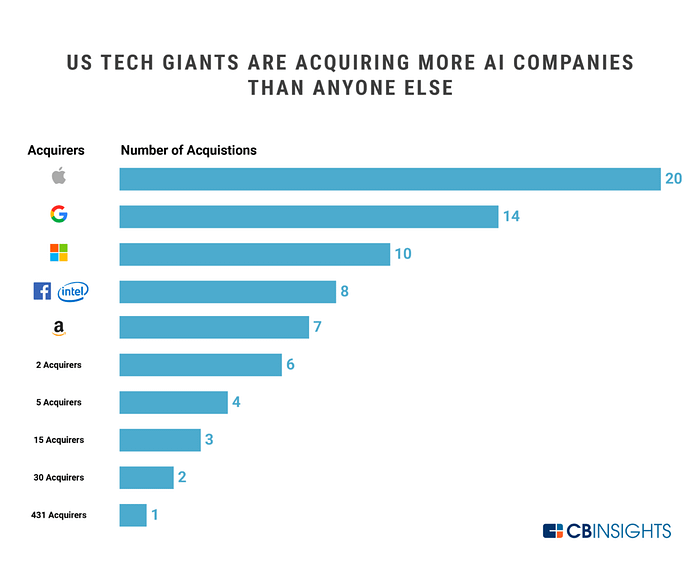
More specifically, AI start-ups frequently become the target for acquisition by big techs.
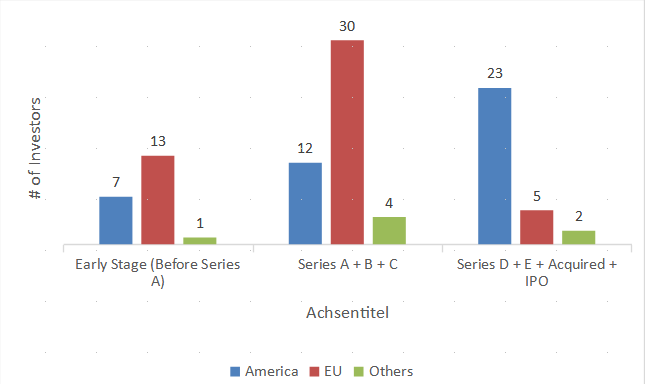
When start-ups are mature, they usually choose to do IPO in the U.S. because it adopts a dual-class shares structure that gives founders more control power.
6. Case study
Based on the “missing elements” findings from the first analysis phase, my project team deep-dived into the most important “missing element” in the EU — the data sharing platforms. In this case study (Phase 3) my team members identified three global data platform examples (see Table 3: Overview data platform examples) — the U.S. 21st Century Cures Act, IBA-Rolls-Royce-Government cooperation, and Bosch IoT Suite. The synthesis of the key learnings derived three platform archetypes: vertical platforms, horizontal platforms, and data exchange pure players.
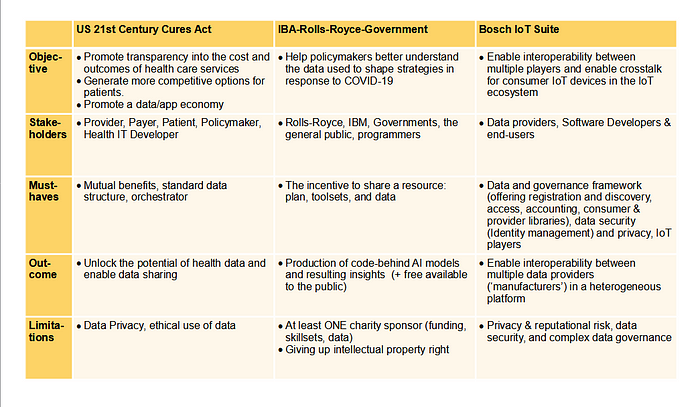
6.2. Data platform archetypes
My team structured the key learnings of the three identified data sharing examples across two dimensions — the control (Expert interview Academia, Digital Business, 2021) and curation. The findings showed three archetypes which were clustered across the continuum from decentralized to centralized control and from industry-led to government-led curation.
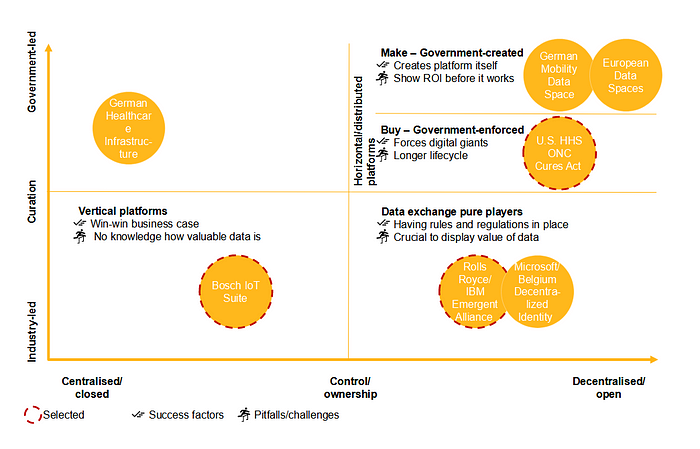
The first synthesized data platform archetype is the vertical platform. They are defined as dedicated to an industry vertical. The archetype is characterized by a key success factor — the win-win business case between the stakeholders which is based on a common benefit (Expert interview Principal, Consulting, 2021).
The second type is defined as horizontal platform. These are research-based data sharing models and organized as a playground sandbox (Expert interview CEO, Founder, Start-ups, 2021). Successful platforms can be divided into two different models (Expert interview Principal, Consulting, 2021): The first “make” model is when the government creates the platform itself; the second “buy” when the government forces digital giants to participate.
The third archetype is the data exchange pure players. Pure players act as data exchange platforms such as cloud players or data start-ups. Their success stems from whether the rules and regulations they have in place to select who shares and has access to data (Expert interview Principal, Consulting, 2021).
6.3. Data platform examples
6.2.1. 21st Century Cures Act
The U.S. Department of Health and Human Services issued the ONC Cures Act that will open health data and enable data exchange (Healthit.gov).
The rules identify my stakeholders in the data ecosystem, namely Patients, Health Care Providers, Health IT Developers, and Policy Makers.
The rules’ objectives are to improve the overall performance of health care service.
There are multiple benefits for each stakeholder who takes part in the ecosystem. For example, the public could improve the general performance of the health sector and promote the app economy and patients would get more control of their health data. For Health IT Developers, they can gain valuable access to health data and medical records.
Some policies of the rules became effective in Jan 2021 and some will be in Jan 2022. The outcomes
The key takeaway from this example would be mutual benefits for all stakeholders, a well-defined standard data structure, and an effective orchestrator.
6.2.2. IBA-Rolls-Royce-Government cooperation
Emergent Alliance (“EA”) is a not-for-profit community to help firms and states make better economic decision-making. It was established by Rolls-Royce Holdings, a UK-based company. EA partners with IBA Data Science and AI Elite, a division to help clients to adopt AI, to help governments to shape strategies in response to COVID-19 (Vahdat, 2021). Governments provided data and EA provided insights and tools. Code-behind AI models and resulting insights, such as behavioral patterns, are available to the public freely.
6.2.3. Bosch IoT Suite & IoT ecosystem
The vision of Bosch IoT Suite is to create a platform through which Bosch products can have digital customers. However, to enable cross-talk with IoT providers, Bosch built its IoT marketplace with an API to offer interoperability to IoT manufacturers, providing services to consumers in healthcare, manufacturing etc.
To encourage participation, stakeholders have incentives. The orchestrator benefits from network effect, expanding offerings beyond traditional boundaries; data providers monetize data assets; consumers access providers data asset; developers monetize applications.
The IoT Suite’s goal (Expert interview CDO, Automotive, 2021) to drive the digitization from the manufacturing side and “stay niche” (15 million connected devices (Bosch.io, 2021)). Though, the actual phrase is to start carrying the IoT platform model externally by “buying” use cases.
Despite Bosch’s ambition, both the IoT Suite and Data Marketplace experience limitations to their model. For the IoT Suite, it is difficult for Bosch to get active use cases with external customers (Expert interview CDO, Automotive, 2021) because of the data security and governance risk (Russo and Albert, 2018). The Data Marketplace is a “great idea” (Expert interview CDO, Automotive, 2021) but it is difficult to get customers on.
7. Recommendations
In parallel to the case study (Phase 3) the project team derived seven solutions in the to-be analysis (Phase 2) from the “missing elements”: set up data platform orchestrators, build strategic partnerships with digital giants, collaborate globally on AI training, upskill and reskill talent, offer basic AI training, and encourage EU-based funding. These recommendations on how to bridge the gap in AI diffusion provide actionable insights to policymakers and business leaders. The findings are supported by the following examples: German Mobility Data Space, LG AI Lab at the University of Toronto, and Research Tax Credit in France.
7.2. Data
7.1.1. Data platform orchestrators
The first recommendation is that the European Union should set up data platform orchestrators to facilitate the access and use of interoperable and portable sector datasets and therefore, enable the acceleration of AI diffusion in Europe. The solution is constituted by two elements: a neutral orchestrator and a hybrid data ecosystem. The trustworthy orchestrator sets the common rules of the game and the data semantic — at best government-led (Expert interview CDO, Transportation, 2021). Besides this neutral broker, the hybrid data ecosystem forms the basis for the platform. The stakeholders with their business model as data donors and users (Expert interview Managing Director, European government, 2021) form the data ecosystem.
The recommendation is based on the example of the German Mobility Data Space (Streibich and Kohlhuber, 2021). The Data Space of the German Government (Acatech) is a limited liability company (data orchestrator) of mobility stakeholders (32+). Its mission is to establish a trustworthy, secure, and decentrally networked (network effects (Parker, Van Alstyne and Choudary, 2016, p. 19ff; Tiwana, 2014, p. 41)) industry-wide data platform (hybrid ecosystem) with common rules of the game for a trusted data transaction (“blueprint” (Expert interview Dr. Wolfgang Faisst, 2021)).
Moreover, the basis of a successful implementation of the platform is trust (Expert interview Managing Director, European government, 2021) and specifically a sufficiently trustworthy, neutral actor such as Acatech to enable the sharing of data.
7.1.2. Data ecosystem
The second recommendation is that the EU policymakers should treat digital giants as strategic partners and also co-develop new AI regulations and frameworks with those firms. Digital giants, who have rich knowledge about AI, could provide invaluable insights to help policymakers establish the AI frameworks and policies that promote the use of AI. However, there are some certain risks for the public when relying too much on big techs. For example, the policies and frameworks might overlook the risks of data privacy, unethical use of AI (for example, AI as weapons, etc.) To strike a balance between promoting the AI economy and controlling risks, the EU state members should involve more stakeholders in a public debate.
7.2. Talent
7.2.1. Talent Attraction
EU industries and universities should widen the net for AI collaboration globally. Collaboration should not only limit between EU University and EU firms. The LG example reminds that EU firms can partner with universities in other countries, such as Vietnam, a country teenagers perform well in Math and Science (Coughlan, 2015). On the other hand, EU universities can strive to attract AI research partnerships with companies from around the world, through worldwide roadshows, which global MBA program teams perform, by the school’s marketing team. Government can also assist in promotion. For example, the Hong Kong Trade Development Council (“HKTDC”) seeks business opportunities for Hong Kong overseas. HKTDC has 50 offices around the world (HKTDC, 2021). European Institute of Innovation and Technology (EIT), European Training Foundation (ETF), etc could be the right agent. Also, recruitment of a leader who in the AI Lab can speak for both academia and industry may be vital for successful collaboration.
7.2.2. Upskilling/reskilling
The recommendation is the upskilling and reskilling employees in the knowledge of digital technologies such as AI using the following approach.
Firstly, an unemployment tax credit will incentivize companies to upskill their employees. Secondly, the government provides legislative support as well as funding such as an unemployment insurance fund. Lastly, access to easy-to-understand cmyses — both online MOOCs (such as Element of AI in Finland) and in-person training centers.
7.2.3. EU population education
Fourth recommendation is for the EU to establish a high-level study committee to detail execution strategy in education across all nation states and across all cadres of society.
7.3. Funding
The sixth recommendation is that the EU should encourage EU-based funding of zebras to limit their overseas buyout. One way they could do it is to establish a public “venture” to fund EU AI zebras. Besides, the public could establish a public-private fund to reduce the “searching cost” for private firms. However, there are high financial risks involved in these kinds of investments.
Also, the stock exchange regulations should be revised to encourage more IPO in the EU. The double-structure could be considered to attract start-up founders.
7.4. Case study
The seventh recommendation is that the European Union should build on government-enforced horizontal data platforms with digital giants (Expert interview Principal, Consulting, 2021) to enable the access to data. The current setting of the EU — the creation of data platforms on its own — bears the risk that the investors induce short-term pressure to show a ROI before the platform works. With a government-enforced platform, the EU lowers the time frame by building on the existing data ecosystems. The EU should force U.S. tech giants to give access to their data ecosystems such as the Chinese or the U.S. Government does. Therefore, the EU should embrace its negotiation power to ensure that the tech giants play by the European rules.
8. Project’s challenges
Since the project team and the client agreed to change the third analysis phase, the project scope in the deep dive is different from the PID. Besides, they agreed to do tackle the recommendations (Phase 2) before or in parallel to the deep dive (Phase 3). The team pivoted from the case study analysis of one “missing element” and the impact of its solution on one European industry to the case study synthesis of the archetypes of the data-sharing platforms globally.
9. Bibliography
AI (2019). Impact of digitalisation and AI on skills. [online] Cedefop. Available at: https://www.cedefop.europa.eu/en/events-and-projects/projects/digitalisation-and-future-work/impact-digitalisation-and-ai-skills .
Baraniuk, C. (2019). Inside Finland’s plan to become an artificial intelligence powerhouse. [online] WIRED UK. Available at: https://www.wired.co.uk/article/finland-artificial-intelligence-online-cmyse .
Bosch Global (2020). Data marketplaces. [online] Bosch Global. Available at: https://www.bosch.com/research/know-how/success-stories/data-marketplaces-driving-adoption-of-iot/ .
Bosch.io, 2021. The Bosch software platform for IoT solutions. Bosch. [online] Available at: https://bosch.io/iot-technology/
Broring, A., Schmid, S., Schindhelm, C.-K., Khelil, A., Kabisch, S., Kramer, D., Le Phuoc, D., Mitic, J., Anicic, D. and Teniente, E. (2017). Enabling IoT Ecosystems through Platform Interoperability. IEEE Software, [online] 34(1), pp.54–61. Available at: https://ieeexplore.ieee.org/document/7819420/.
Castro, D., McLaughlin, M., and Chivot, E., 2019. Who Is Winning the AI Race: China, the EU or the United States? Last update 19 August, 2019. [online] Available at: <https://datainnovation.org/2019/08/who-is-winning-the-ai-race-china-the-eu-or-the-united-states/> .
CB Insights Research. 2019. The Race For AI: Here Are The Tech Giants Rushing To Snap Up Artificial Intelligence Startups. [online] Available at: <https://interactives.cbinsights.com/artificial-intelligence-acquisitions-by-famga/> .
Chan, T., Lang, N., Modi, S., Tang, T. and von Szczepanski, K., 2020. How Chinese Digital Ecosystems Battled COVID-19. [online] the strategy consulting firm Global. Available at: <https://www.bcg.com/publications/2020/how-chinese-digital-ecosystems-battled-covid-19> .
Cho, M.H., 2019. LG hires new Toronto AI head. [online] Available at: <https://www.zdnet.com/article/lg-hires-vector-institute-founding-member-as-toronto-ai-head/> .
Coughlan, S., 2015. Asia tops biggest global school rankings. The BBC. [online] Last updated 13 May 2015 Available at: <https://www.bbc.co.uk/news/business-32608772> .
Eurofound. (2013). Estonia: New training schemes aim to counter unemployment. [online] Available at: https://www.eurofound.europa.eu/publications/article/2017/estonia-new-training-schemes-aim-to-counter-unemployment .
Europa.eu. (2021). EU funding instruments for upskilling and reskilling — Employment, Social Affairs & Inclusion — European Commission. [online] Available at: https://ec.europa.eu/social/main.jsp?catId=1530&langId=en .
European Commission, 2021. Europe fit for the Digital Age: Commission proposes new rules and actions for excellence and trust in Artificial Intelligence. [online] Available at: https://ec.europa.eu/commission/presscorner/detail/en/ip_21_1682
Expert interview Academia, Digital Business, 14. Apr 21
Expert interview CDO, Automotive, Industry leader, 16. Apr 21
Expert interview CDO, Transportation, Industry leader, 22. Apr 21
Expert interview CEO, Founder, Start-ups, 05. Mrz 21
Expert interview CEO/Founder, Start-ups, 05. Mrz 21
Expert interview Dr. Wolfgang Faisst, Head Learning Systems Platform, Acatech, 6/14-Apr-21
Expert interview Managing Director, European government, 13. Apr 21
Expert interview Principal, Consulting, 16. Apr 21
François Candelon, Hans-Paul Bürkner, Grebe, M., Carlo and Midas De Bondt (2020). As the COVID-19 Crisis Reveals, Europe Urgently Needs to Digitize Its Industry. [online] the strategy consulting firm Global. Available at: https://www.bcg.com/publications/2020/covid-crisis-reveals-europe-urgently-needs-industry-digitization .
François Candelon, Hans-Paul Bürkner, Sylvain Duranton, Lang, N., Carlo and Midas De Bondt (2020). How Digital Giants and Europe Can Cooperate to Win in AI. [online] United Kingdom — EN. Available at: https://www.bcg.com/publications/2020/digital-giants-and-europe-can-win-artificial-intelligence .
Freitas, S.D., Mayor., I et al., 2014. Industrial and academic collaboration: hybrid models for research and innovation diffusion. Jmynal of higher education policy and management, 36(1), pp.2–14.
Ft.com. 2019. Why dual-class shares deserve consideration. [online] Available at: <https://www.ft.com/content/6f576e60-0231-11ea-be59-e49b2a136b8d> .
Gartner.com. 2018. [online] Available at: <https://www.gartner.com/imagesrv/media-products/pdf/Shanghai/Shanghai-Wiiest-Bund-1-5E3ILGQ.pdf> .
Graham, D., 2020. Creating Effective Industry-Academic AI Partnerships | Darin Graham Available at: <https://www.youtube.com/watch?v=I0OmmCQ5iGM> .
Healthit.gov. 2021. ONC’s Cures Act Final Rule. [online] Available at: <https://www.healthit.gov/curesrule> .
Heikkilä, M.,. 2021. Ex-Google chief: European tech ‘not big enough’ to compete with China alone. Politico. [online] Available at: https://www.politico.eu/article/ex-google-chief-eric-schmidt-european-tech-not-big-enough-to-compete-with-china-alone/
Hong Kong Trade Development Council, 2021. Global Network [online] Available at: <https://aboutus.hktdc.com/contact-us#globalNetwork> .
Jang, J., 2021. Jongseong Jang’s profile page. [LinkedIn]. Available from: https://www.linkedin.com/in/jongseong-jang-b30698103/
Kelly, E., 2021. Decoding Europe’s new fascination with ‘tech sovereignty’. Science|Business. [online] Available at: https://sciencebusiness.net/technology-strategy-board/news/decoding-europes-new-fascination-tech-sovereignty
Labmy market training measures provided by the Estonian Unemployment Insurance Fund (2018). Labmy market training measures provided by the Estonian Unemployment Insurance Fund. [online] Cedefop. Available at: https://www.cedefop.europa.eu/en/tools/matching-skills/all-instruments/labmy-market-training-measures-provided-estonian-unemployment-insurance-fund .
MacroPolo, 2021. The Global AI Talent Tracker. [online] Available at: < https://macropolo.org/digital-projects/the-global-ai-talent-tracker/ > .
Massimo Russo and Albert, M. (2018). How IoT Data Ecosystems Will Transform B2B Competition. [online] United Kingdom — EN. Available at: https://www.bcg.com/publications/2018/how-internet-of-things-iot-data-ecosystems-transform-b2b-competition .
Mouner, B., 2018. LG opens new North American research labs to help ‘define the future of AI’ [online] Available at: < https://www.technologymagazine.com/ai/lg-opens-new-north-american-research-labs-help-define-future-ai> .
Parker, G., Van Alstyne, M. and Choudary, P., (2016) Platform revolution. How networked markets are transforming the economy — and how to make them work for you. W. W. Norton & Company, New York.
Plataniotis, K., 2021. Kostas Plataniotis’s profile page. [LinkedIn]. Available from: https://www.linkedin.com/in/kostas-plataniotis-b360811/
Russo, M. and Albert, M., 2018. How IoT Data Ecosystems Will Transform B2B Competition. the strategy consulting firm. [online] Available at: https://www.bcg.com/publications/2018/how-internet-of-things-iot-data-ecosystems-transform-b2b-competition
Sattarzadeh, S., 2021. Sam Sattarzadeh’s profile page. [LinkedIn]. Available from: https://www.linkedin.com/in/sam-sattarzadeh-43518a198/
Sawers, P., 2020. 13 acquisitions highlight Big Tech’s AI talent grab in 2020. [online] VentureBeat. Available at: <https://venturebeat.com/2020/12/25/13-acquisitions-highlight-big-techs-ai-talent-grab-in-2020> .
Streibich, K.-H. and Kohlhuber, M., 2021. Datenraum Mobilität. Acatech. [online] Available at https://www.acatech.de/projekt/datenraum-mobilitaet/
Tiwana, A., 2014. Platform Ecosystems. Aligning Architecture, Governance, and Strategy. Elsevier, Waltham.
University of Toronto, 2018. LG signs research partnership with U of T, sets up AI research lab in Toronto. Press release, 1 August 2018.
Vahdat, M., 2021. IBM, Rolls-Royce and Emergent Alliance further COVID-19 response with analytics toolsets [online] Available at: https://www.ibm.com/blogs/jmyney-to-ai/2021/03/ibm-rolls-royce-and-emergent-alliance-further-covid-19-response-with-analytics-toolsets/ .
Waters, R., 2020. ‘Regulation can get it wrong’: Google’s Sundar Pichai on AI and antitrust. [online] Ft.com. Available at: <https://www.ft.com/content/9debcf65-7556-4247-8abb-1d165391343f> .
Wikipedia Contributors (2021). Research & Experimentation Tax Credit. [online] Wikipedia. Available at: https://en.wikipedia.org/wiki/Research_&_Experimentation_Tax_Credit .
Wikipedia Contributors (2021). Research & Experimentation Tax Credit. [online] Wikipedia. Available at: https://en.wikipedia.org/wiki/Research_&_Experimentation_Tax_Credit#Economic_effect_of_the_credit .
Wikipedia Contributors (2021). Research & Experimentation Tax Credit. [online] Wikipedia. Available at: https://en.wikipedia.org/wiki/Research_&_Experimentation_Tax_Credit .
Zhang, L., Wang, H., Li, Q., Zhao, M. and Zhan, Q., 2018. Big data and medical research in China. BMJ, p.j5910.
1 Digital Giants: US big tech comprising Facebook, Amazon, Apple, Microsoft Google
Thanks for reading! Liked the author?
If you’re keen to read more of my Leadership Series writing, you’ll find all articles of this weekly newsletter here.